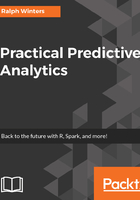
Comparing a sample to the population
To illustrate some of the benefits of sampling, and to see how you can often get close to the same results with a sample as with a larger population, copy the following code and run it within an R script. This script will generate a 15,000,000 row population and then extract a 100-row random sample. Then we will compare the results:
large.df <- data.frame(
gender = c(rep(c("Male", "Female", "Female"), each = 5000000)),
purchases = c(0:9, 0:5, 0:7)
)
#take a small sample
y <- large.df[sample(nrow(large.df), 100), ]
mean(large.df$purchases)
mean(y$purchases)
#Render 2 plots side-by-side by setting the plot frame to 1 by 2
par(mfrow=c(1,2))
barplot(table(y$gender)/sum(table(y$gender)))
)barplot(table(large.df$gender)/sum(table(large.df$gender))
#Return the plot window to a 1 by 1 plot frame
par(mfrow = c(1, 1))
Switch over to the console and note that the sample mean is close to the population mean:
> mean(large.df$purchases)
[1] 3.666667
> mean(y$purchases)
[1] 3.64
Now, switch over to the plot area and note that the distribution of gender is also similar when comparing the sample (left plot), and the population (right plot):

I encourage you to take some large datasets on your own, practice taking some random samples and seeing how close you get to population results. Possibly that will enable you to work faster and in a more productive manner.