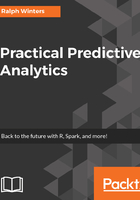
Using all of the data
However, many data scientists will choose to use the entire population as the basis of analysis, instead of a sample. I suspect one reason is that often it is difficult to obtain a reliable sample, especially when the data has many different sources, and some are of unknown data quality. Good reliable data costs money to collect. Another reason is that the data scientists may not be familiar with sampling techniques, or believe that more is always better. Therefore, it is important to understand some important points about using all of the data:
- You can use all of the data if it accurately represents the underlying population. If all the data is the underlying population, you can't do better than that, but proceed with caution. What you think is a population may just be a very large sample. Future data may reflect a reality that is completely different. For very large data sets, you can never be quite sure if what we are looking at IS representative, since the amount of data can simply be overwhelming, and the underlying data collection methods may be unknown or unreliable. Be particular careful for data which is collected over long periods of time. Often data collection methods change, or the way calculations are performed are altered.
- When you use an incredibly large amount of data, what you are measuring is not necessarily representative of the factors which motivate the response. For example, clickstream data and online survey data is not always representative of the why? Deeper inspection (through smaller samples) is always advisable to examine motivation.
- As mentioned earlier, processing huge amounts of data consumes a lot of computational resources. Processing accurate samples can take a fraction of that time
- With large datasets, you will just about always find correlations somewhere. Related to this is the concept of significant correlation versus effect size. This means that even if you find a statistically significant correlation, the differences can be so slight, so as to render any association meaningless or nonactionable.
So the first line of attack for this is to try to understand how the data was collected. It may be biased towards certain age groups, genders, technology users, and so on. There may be ways to fix this using a technique known as oversampling in which you weigh certain under-represented groups in a way which more realistically represents their frequency.