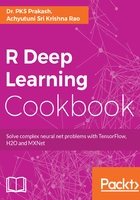
上QQ阅读APP看书,第一时间看更新
How it works...
The performance of the model can be evaluated using AUC:
# Performance on Train
library(pROC)
ypred <- sess$run(tf$nn$sigmoid(tf$matmul(x, W) + b))
roc_obj <- roc(occupancy_train[, yFeatures], as.numeric(ypred))
# Performance on test
nRowt<-nrow(occupancy_test)
xt <- tf$constant(unlist(occupancy_test[, xFeatures]), shape=c(nRowt, nFeatures), dtype=np$float32)
ypredt <- sess$run(tf$nn$sigmoid(tf$matmul(xt, W) + b))
roc_objt <- roc(occupancy_test[, yFeatures], as.numeric(ypredt)).
AUC can be visualized using the plot.auc function from the pROC package, as shown in the screenshot following this command. The performance for training and testing (hold-out) is very similar.
plot.roc(roc_obj, col = "green", lty=2, lwd=2)
plot.roc(roc_objt, add=T, col="red", lty=4, lwd=2)

Performance of logistic regression using TensorFlow