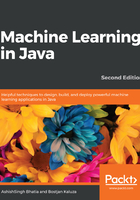
Apache Spark
Apache Spark, or simply Spark, is a platform for large-scale data processing builds atop Hadoop, but, in contrast to Mahout, it is not tied to the MapReduce paradigm. Instead, it uses in-memory caches to extract a working set of data, process it, and repeat the query. This is reported to be up to ten times as fast as a Mahout implementation that works directly with data stored in the disk. It can be grabbed from https://spark.apache.org.
There are many modules built atop Spark, for instance, GraphX for graph processing, Spark Streaming for processing real-time data streams, and MLlib for machine learning library featuring classification, regression, collaborative filtering, clustering, dimensionality reduction, and optimization.
Spark's MLlib can use a Hadoop-based data source, for example, Hadoop Distributed File System (HDFS) or HBase, as well as local files. The supported data types include the following:
- Local vectors are stored on a single machine. Dense vectors are presented as an array of double-typed values, for example, (2.0, 0.0, 1.0, 0.0), while sparse vector is presented by the size of the vector, an array of indices, and an array of values, for example, [4, (0, 2), (2.0, 1.0)].
- Labelled point is used for supervised learning algorithms and consists of a local vector labelled with double-typed class values. The label can be a class index, binary outcome, or a list of multiple class indices (multiclass classification). For example, a labelled dense vector is presented as [1.0, (2.0, 0.0, 1.0, 0.0)].
- Local matrices store a dense matrix on a single machine. It is defined by matrix dimensions and a single double-array arranged in a column-major order.
- Distributed matrices operate on data stored in Spark's Resilient Distributed Dataset (RDD), which represents a collection of elements that can be operated on in parallel. There are three presentations: row matrix, where each row is a local vector that can be stored on a single machine, row indices are meaningless; indexed row matrix, which is similar to row matrix, but the row indices are meaningful, that is, rows can be identified and joins can be executed; and coordinate matrix, which is used when a row cannot be stored on a single machine and the matrix is very sparse.
Spark's MLlib API library provides interfaces for various learning algorithms and utilities, as outlined in the following list:
- org.apache.spark.mllib.classification: These are binary and multiclass classification algorithms, including linear SVMs, logistic regression, decision trees, and Naive Bayes
- org.apache.spark.mllib.clustering: These are k-means clustering algorithms
- org.apache.spark.mllib.linalg: These are data presentations, including dense vectors, sparse vectors, and matrices
- org.apache.spark.mllib.optimization: These are the various optimization algorithms that are used as low-level primitives in MLlib, including gradient descent, stochastic gradient descent (SGD), update schemes for distributed SGD, and the limited-memory Broyden–Fletcher–Goldfarb–Shanno (BFGS) algorithm
- org.apache.spark.mllib.recommendation: These are model-based collaborative filtering techniques implemented with alternating least squares matrix factorization
- org.apache.spark.mllib.regression: These are regression learning algorithms, such as linear least squares, decision trees, Lasso, and Ridge regression
- org.apache.spark.mllib.stat: These are statistical functions for samples in sparse or dense vector format to compute the mean, variance, minimum, maximum, counts, and nonzero counts
- org.apache.spark.mllib.tree: This implements classification and regression decision tree-learning algorithms
- org.apache.spark.mllib.util: These are a collection of methods used for loading, saving, preprocessing, generating, and validating the data