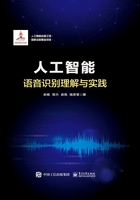
4.2 使用误差反向传播进行参数训练
从20世纪80年代开始,人们知道了有着足够大隐层的多层感知器(Multi-Layer Perceptron,MLP)是一个通用的近似算子(Universal Approximator)[183]。换句话说,有足够大隐层的MLP可以近似任意一个从输入空间。显然,既然DNN是多隐层的MLP,那么它自然可以作为一个通用近似算子。
DNN的模型参数{W, b}需要通过每个任务的训练样本|0≤m<M}来训练得到,其中M是训练样本个数,om是第m个观察向量,ym是对应的输出向量。这个过程被称为训练过程或者参数估计过程,需要给定一个训练准则和学习算法。
4.2.1 训练准则
训练准则应该能够被简单地计算,并且与任务有很高的相关性,准则上的提升最后应该能体现到任务的完成水准上。在理想情况下,模型参数的训练应该最小化期望损失函数

其中,J(W, b; o, y)是损失函数,{W, b}是模型参数,o是观察向量,y是相应的输出向量,p(o)是概率密度函数。不幸的是,p(o)需要从训练集中估计,对训练集中没有出现的样本J(W, b; o, y)也没有被很好地定义(集外样本的标注是未知的)。因此,DNN往往采用经验性准则来训练。
在DNN训练中有两个常用的训练准则。对于回归任务,均方误差(Mean Square Error,MSE)准则

经常被使用,其中

对分类任务,设y是一个概率分布,那么交叉熵(Cross Entropy,CE)准则

经常被使用,其中

yi=Pemp(i|o)是观察o属于类i的经验概率分布(从训练数据的标注中来),是采用DNN估计的概率。最小化交叉熵准则等价于最小化经验分布和DNN估计分布的KL距离(Kullback-Leibler Divergence,KLD)。一般来说,人们通常使用硬标注来描述经验概率分布,即
,其中

是指示函数,c是训练集对于观察o的标注类别。在大部分情况下,公式(4.11)下的CE准则退化为负的对数似然准则(Negative Log-likelihood,NLL)

4.2.2 训练算法
给定训练准则,模型参数{W, b}可以使用著名的误差反向传播(Backpropagation,BP)算法[47]来学习,可以使用链式法则来推导。[2]
在其最简单的形式下,模型参数使用一阶导数信息按照如下公式来优化:

其中,分别是在第t次迭代更新之后ℓ层的权重矩阵(Weight Matrix)和偏置向量(Bias Vector)。

以上分别是在第t次迭代时得到的平均权重矩阵梯度和平均偏置向量梯度,这些是使用Mb个训练样本得到的,ε是学习率,∇xJ是J相对x的梯度。
顶层权重矩阵相对于训练准则的梯度取决于训练准则。对于回归问题,当MSE训练准则(公式(4.9))和线性输出层(公式(4.5))被使用时,输出层权重矩阵的梯度是

我们定义输出层的误差信号为

类似地,

对于分类任务,CE训练准则(公式(4.11))和softmax输出层(公式(4.6))被使用,输出层权重矩阵的梯度为

类似地,我们定义输出层的误差信号为

类似地,

注意,(公式(4.21))看上去与
(W, b; o, y)有相同的形式(公式(4.18))。不过,因为做回归时
,而做分类时
,所以它们其实是不同的。
对于0<ℓ<L,则有

其中,是层ℓ的误差信号,•是元素级相乘,diag(x)是一个对角线为x的方矩形,
是激活函数的元素级导数。对于sigmoid激活函数来说,则有

类似地,tanh激活函数的导数为

其中,每个元素的符号函数。误差信号能从顶层向下反向传播

对于ℓ<L,则有

反向传播算法的关键步骤在算法4.2中进行了总结。