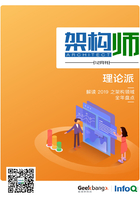
特别专栏 | Column
在ARM盒子上使用IEF和Tensorflow Lite运行边缘AI

场景介绍
在ARM盒子(我使用atlas500, arm64v8架构可以直接使用代码和镜像)上,接入网络摄像头,使用tensorflow lite框架,对图像进行图像分类推理,图像分类推理结果通过MQTT协议推送到web页面上进行展示。参考“在ARM盒子使用IEF和Tensorflow运行边缘AI”,这次使用tensorflow lite框架,并把对象检测模型更换成图像分类模型。

运行环境
· 网络摄像头,支持RTSP协议,ipc格式
· 智能小站(atlas500),EulerOS
· tensorflow1.4.0, python2.7
· mosquitto 1.6.5
· python: opencv-python 4.1.1, paho-mqtt 1.4.0
· nodejs: mqtt 3.0.0, react 16.9.0
安装运行步骤
参考“在ARM盒子上使用IEF和Tensorflow运行边缘AI”,本文仅更换成tensorflow lite框架,并使用图像分类模型。
通过tensorflow对图像进行图像分类推理
先从
https://tensorflow.google.cn/lite/models/image_classification/overview
下载图像分类模型
并解压出模型和分类标签。
参考
代码,添加opencv对rtsp协议进行解析,并获取每一帧图像。针对图像数据,运行模型推理进行图像分类。最终推理的结果使用MQTT协议把结果发送出来。
参考代码-----如下:

完整的docker image可以在docker hub上参考
braveyuyong/tf_on_atlas:tagname:0.2.1-lite,
参考dockerfile如下:

最终效果

参考"在ARM盒子使用IEF和Tensorflow运行边缘AI"中下发应用,最终通过浏览器IE11打开http://192.168.1.111:3001,如果能看到摄像头视频流和图像分类结果,表明大功告成!